According to Lingaro Senior Data Scientist and AI Advisor Maciej Michalek, the biggest potential of large language models (LLMs) lies in its capacity to save valuable time and workers’ effort. Yet, after the initial hype of ChatGPT, a lot of managers still haven’t realized how much more efficient their operations can be by using LLMs for certain tasks. Michalek says that once business leaders see what they’re missing, LLMs will empower whole new industries.
A large language model is a type of generative AI algorithm that uses massively huge text-based data sets to understand existing text and generate brand new text. Phrased differently, an LLM’s features are natural language understanding and natural language generation. This tool has many business use cases, which we’ll get into in a bit. Despite this, a business can still be apprehensive about adopting LLMs given steep upgrade costs and difficulties in establishing a data culture across the entire organization.
Another reason may be that they don’t know that they want this technology yet, and that it’s up to IT service providers to show them what they want. In its bid to meaningfully compete with ChatGPT, Google released Gemini LLM, which is apparently five times more powerful than the former’s LLM, GPT-4. More power generally means more capability, speed, and reliability, which are what all businesses want from their tools.
Primary capabilities and benefits of LLMs
In business settings, LLMs have the following primary capabilities and benefits:
Capabilities | Benefits |
Automatically summarize pieces of text, such as internal documents, and highlight key information from them | No need to have people manually read through so many documents |
Accurately lift relevant information from large volumes of documents to include in reports | Avoids manual encoding errors |
Enables text search in internal knowledge databases | Allows users to ask questions like “What were the top-selling SKUs per region last month?” and get an accurate answer. |
Generate new text, such as automated email responses, product descriptions, and reports | Saves staff time and effort |
Write code | Accelerates the development process and makes it less costly |
The current poster child of LLMs is arguably the conversational AI chatbot. Its predecessor, the scripted chatbot, already helps millions of customers via preset questions and answers. Conversational AIs promise to be even more helpful by beginning chat engagements with open-ended questions like “How can I help you?” This lets people type up their concerns directly without having to parse through multiple option menus. They’ll also be more likely to elaborate on what they want and provide details they wouldn’t be able to give if they’re limited to stock answers. Moreover, since conversations are less structured and more natural sounding, they’ll feel like they are talking with another person and become more at ease compared to when they know that they’re talking with a robot. In the near future, we’ll audibly talk with chatbots, if robots Desdemona and Sophia have anything to say about it.
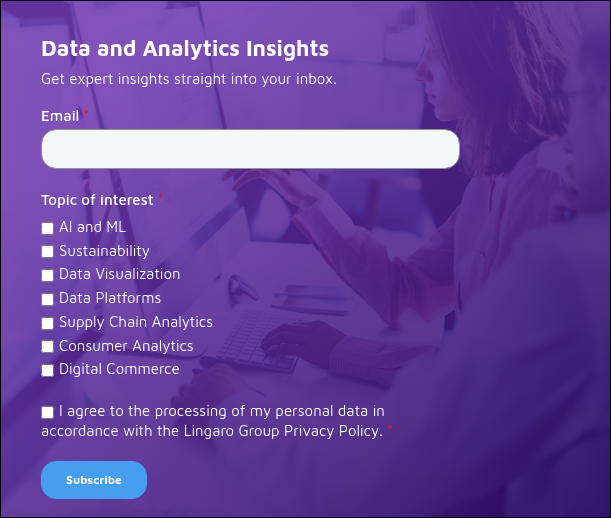
LLM and RPA augment one another
Another way of using LLMs that’s growing in popularity is augmenting robotic process automation (RPA). Separately, RPA bots executes programmed workflows, and LLMs understand and respond with natural language. However, both technologies can be combined to work in two ways. The first way is by adjusting LLMs so that they create output that RPA bots can use. For instance, LLMs can be used to parse through and extract key information from invoices or even inconsistently written email orders. An RPA bot can then use the extracted information to populate relevant databases and process orders.
The second way is by having an RPA bot gather information for an LLM to generate documents with. For example, an RPA bot can gather accounting and financial information from multiple sources within the company’s database. The LLM can then generate comprehensive accounting statements and readable financial reports.
Other business use cases for LLM-RPA hybrids include sentiment analysis and sales support. In sentiment analysis, an LLM analyzes feedback and comments on social media to gauge if customers feel positive, negative, or neutral about a product or service. The RPA then collates the info into insightful reports.
In sales support, an RPA tracks customer behavior and product choices to identify that person’s preferences and assign them a score pertaining to how likely they are to make a purchase. An LLM can then generate personalized sales emails and product recommendations.
Being able to talk with our computers is awesome
"Thanks to LLMs, a lot of tasks will be automated,” Michalek says. “LLMs will help doctors to prepare diagnoses and treatment plans faster. Lawyers will have a nice tool that not only helps them draft agreements but also looks for the best line of argumentation in lawsuits. Lots of help desk tasks will be fully automated. LLMs will also revolutionize the search process, as it can merge information from multiple sources into valuable and expected insights.”
He also shared that a generative AI model that’s geared for data visualization can get a huge boost from LLMs. “We’ll just ask a chatbot what we need in our business report using natural language, and we’ll get a report that’s well-visualized in response.” Once we’ve pushed LLMs farther — as we’ll undoubtedly do — we’ll soon be able to talk with a robot and tell it what we want, and it’ll do it.