Producing business insights from multivarious analytics tools is one thing, but turning those insights into actual business value is another. In fact, Gartner’s 2023 CDO survey revealed that 69% of Data & Analytics leaders are finding it hard to deliver quantifiable return on investment (ROI) from their initiatives. In this article, we will show how some blockers to value creation are data governance problems, and that strategies that are based on a grounded and business-oriented approach are effective in removing those blockers.
According to Google, data governance is “everything you do to ensure data is secure, private, accurate, available, and usable. It includes the actions people must take, the processes they must follow, and the technology that supports them throughout the data life cycle.” Data governance is a form of centralized data management that is principled. It complies with standards imposed by stakeholders, government agencies, and industry associations. It also follows internally set standards or data policies for how data is collected, kept, used, and disposed of. For example, a policy can set what type of personnel can access a certain type of data.
Since we’re discussing ROI, it seems counterintuitive to tack on hefty data governance costs into the equation. However, if people think that compliance is expensive, wait ‘till they try non-compliance.
Poor data governance impedes value realization
Without proper data governance, enterprises face many challenges, such as lack of data consistency; difficulties in upholding data security, privacy, and regulatory requirements; the formation of data silos; and the lack of data observability, which creates the need for repeated checks and validations prior to using data.
Lack of data consistency
In the creation and use of data systems and analytics tools, stakeholders, such as business units, data engineers, developers, and data users must agree on the terms of data curation. These terms include what data is gathered from where, how the data is standardized, formatted, and understood, and what level of quality is acceptable for data to be ingested. If data scientists acquire the data sets that do not match what business users require, then the tools built with these would provide results that users don’t need or deem to be untrustworthy.
Another problem lies in failing to establish well-defined data ownership. Data ownership refers to both who has the right to control, use, and manage a specific set of data, as well as who is responsible for ensuring its security, quality, and compliance with standards and regulations. On a basic level, knowing who owns the data means that stakeholders know whom to ask access rights from. At a higher level, knowing where a piece of data came from allows users to understand its context, spot potential biases in it, and determine if it’s valid and could be reliably used for making decisions. At a granular level, well-defined data ownership could contribute to efficient data management. To illustrate, some data sets are aggregated from different sources and may involve compliance with regulations from different jurisdictions, so having clear data ownership at the start could mean processing compliance from day one or day 60.
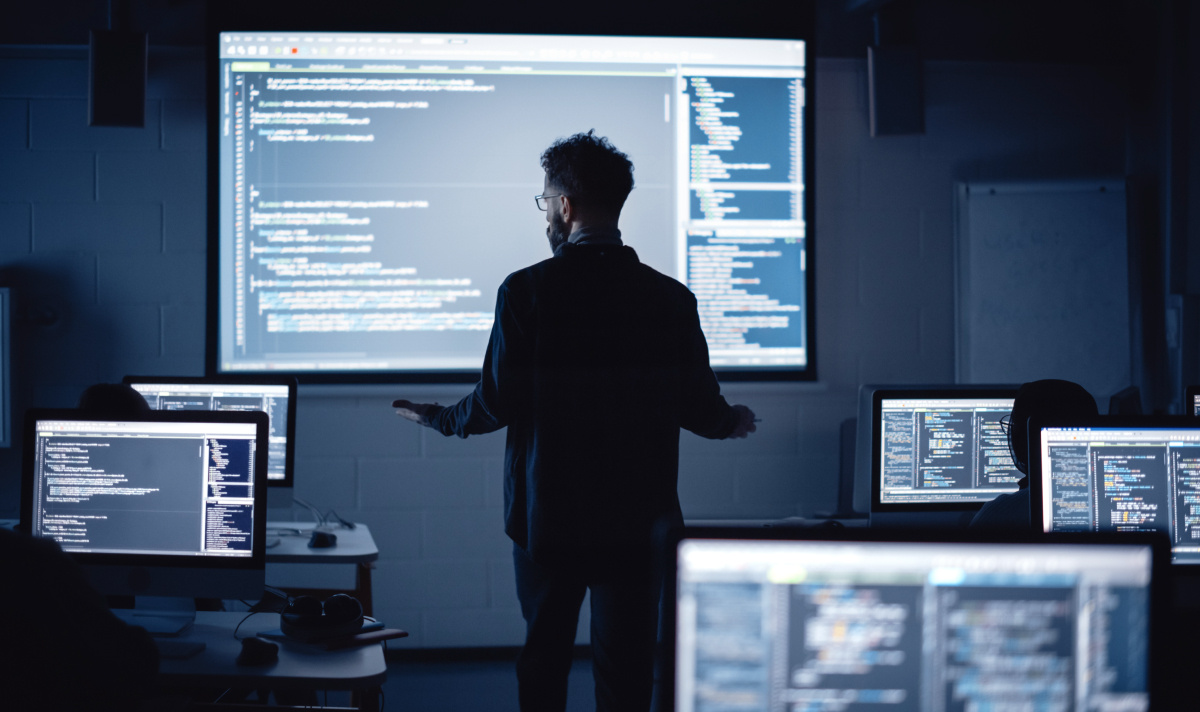
Data governance strategies for amplifying analytics ROI:
- Have stakeholder agree on the terms of data curation.
- Establish well-defined data ownership.
Difficulties in delivering data security and privacy, as well as meeting regulatory requirements
Without good security protocols in place, data could be easily stolen, tainted, corrupted, or deleted, which could then cause analytics to produce erroneous, misleading, and unreliable findings and insights.
Having weak privacy protections could lead to data breaches and misappropriations of personal and sensitive information. These, in turn, could lead to identity theft and to the misuse of analytics on individuals. To illustrate, personal data that is meant for academic research could be processed by marketing tools to discern the types of advertising that would be most effective for certain types of individuals. Personal data insecurity breeds consumer distrust and gives offending firms a bad reputation.
To remain trustworthy, the least that companies can do is to comply with data regulations. Failing to do so may result in being penalized with administrative fines or suspension of company operations. A more holistic method for creating transparency and trust involves implementing data oversight protocols, such as data audits and privacy and security checks.
Data governance strategy for amplifying analytics ROI:
Implement strong, consistent, and compliant data oversight protocols across the entire organization.
Formation of data silos
Operational inefficiencies arise when data sources are not contextually integrated or shared with other analytical systems. Departments could have different formats for the same data, making their data difficult to integrate for analytics purposes. It’s also likely that different teams would end up integrating with incorrect business context, thereby wasting time and effort. Perhaps they’d also waste processing power and storage space if their projects were big enough.
Moreover, silos have their own access configurations, which means that pulling information from different areas of the company would be a time-consuming process. Research projects would therefore take much slower than if permissions were centrally managed. Finally, silos tend to have their own security standards and measures, which means that there would be some that are more lax. In those silos, data users could be using risky applications and storing data in unsecure locations, thereby exposing the data to greater risk of being breached.
Data governance strategy for amplifying analytics ROI:
Break down data silos and centralize data management to streamline processes and standardize protocols.
Lack of data observability
No information system is perfect. Data inconsistencies might arise in some data pipelines, while the reliability and quality of data might drop in others. Without data observability, data managers won’t have clarity as to what’s going wrong with their data, much less form strategies to resolve those issues. Moreover, if the enterprise tries to scale its analytics and AI tools and the data is of poor quality, then the tools would produce incorrect analyses and insights, which would defeat the purpose of analytics systems in competitive industries.
With data observability, data managers gain a clear view of data flows so that they can keep data accurate and valid. They can also spot and fix data issues in real time, which means that the quality and reliability of the output of analytics tools could be maintained.
Data governance strategy for amplifying analytics ROI:
Establish data observability across the entire data ecosystem.
A slightly different approach to data governance
Lingaro is like other data service providers in that it does implement industry standards like the ones set in the Data Management Body of Knowledge (DMBoK 2) by the Data Management Association (DAMA). However, there are three aspects of Lingaro’s approach to data governance that help organizations readily achieve greater analytics ROI through data governance strategies.
The additional step of consulting the business
While other approaches to data governance might focus on the technological side of data governance solutions, Lingaro always takes care to first learn about the business side of the enterprise to understand its data strategy, its current data governance capabilities, and its data governance needs as they relate to the outcome of overall strategy.
In this step, it is important to establish standard definitions of success for both the business side and the IT side of things. One such common definition of success could be the significant increase in the business value created by the various investments in analytics.
Non-invasive data governance program
Popular approaches — especially the ones that focus on the technological side of data governance solutions — espouse an invasive program (coined by Robert S. Seiner) wherein data systems are overhauled to accommodate the solutions. This drastic change can prove to be expensive, time-consuming, and difficult to adapt to. Our approach is focused on comprehensible, concise and nimble data governance solutions.
Lingaro, however, follows the non-invasive data governance program framework to provide value without having to initiate the program from scratch. The approach involves assessing the existing program and placing the set of processes under the right track to scale. In practice, data management and data quality processes and standards are commonly adhered to within the organization without being named officially under data governance function. So, our approach simply realigns these processes and standards with data governance goals. Specifically, our non-invasive approach entails the following:
- Strategic realignment of sequence of activities with existing data governance functions to enhance efficiency, agility and scalability.
- Model assessment, prioritization of best working models, and the extension of similar models to solve critical issues and benefit the program.
- Awareness, training, and implementation of data governance knowledge across functions to increase adoption and eventually improve data governance processes.
Specialization in Data Governance
There are different standards and regulations that govern your data, depending on where that data resides. Moreover, over time, new laws are introduced, and old ones are amended. For enterprises operating in multiple territories, compliance is a headache — unless it is partnered with a firm like Lingaro. Lingaro’s Data Governance Team always keeps up with government and industry regulations to ensure that clients are compliant.
Lingaro’s data governance specialists handle all sorts of challenges that multinational enterprises face. Contact us for a discovery call or a data governance maturity assessment.